Joachim Breitner's Homepage
Blog
Do surprises get larger?
The setup
Imagine you are living on a riverbank. Every now and then, the river swells and you have high water. The first few times this may come as a surprise, but soon you learn that such floods are a recurring occurrence at that river, and you make suitable preparation. Let’s say you feel well-prepared against any flood that is no higher than the highest one observed so far. The more floods you have seen, the higher that mark is, and the better prepared you are. But of course, eventually a higher flood will occur that surprises you.
Of course such new record floods are happening rarer and rarer as you have seen more of them. I was wondering though: By how much do the new records exceed the previous high mark? Does this excess decrease or increase over time?
A priori both could be. When the high mark is already rather high, maybe new record floods will just barley pass that mark? Or maybe, simply because new records are so rare events, when they do occur, they can be surprisingly bad?
This post is a leisurely mathematical investigating of this question, which of course isn’t restricted to high waters; it could be anything that produces a measurement repeatedly and (mostly) independently – weather events, sport results, dice rolls.
The answer of course depends on the distribution of results: How likely is each possible results.
Dice are simple
With dice rolls the answer is rather simple. Let our measurement be how often you can roll a die until it shows a 6. This simple game we can repeat many times, and keep track of our record. Let’s say the record happens to be 7 rolls. If in the next run we roll the die 7 times, and it still does not show a 6, then we know that we have broken the record, and every further roll increases by how much we beat the old record.
But note that how often we will now roll the die is completely independent of what happened before!
So for this game the answer is: The excess with which the record is broken is always the same.
Mathematically speaking this is because the distribution of “rolls until the die shows a 6” is memoryless. Such distributions are rather special, its essentially just the example we gave (a geometric distribution), or its continuous analogue (the exponential distributions, for example the time until a radioactive particle decays).
Mathematical formulation
With this out of the way, let us look at some other distributions, and for that, introduce some mathematical notations. Let X be a random variable with probability density function φ(x) and cumulative distribution function Φ(x), and a be the previous record. We are interested in the behavior of
Y(a) = X − a ∣ X > x
i.e. by how much X exceeds a under the condition that it did exceed a. How does Y change as a increases? In particular, how does the expected value of the excess e(a) = E(Y(a)) change?
Uniform distribution
If X is uniformly distributed between, say, 0 and 1, then a new record will appear uniformly distributed between a and 1, and as that range gets smaller, the excess must get smaller as well. More precisely,
e(a) = E(X − a ∣ X > a) = E(X ∣ X > a) − a = (1 − a)/2
This not very interesting linear line is plotted in blue in this diagram:
The orange line with the logarithmic scale on the right tries to convey how unlikely it is to surpass the record value a: it shows how many attempts we expect before the record is broken. This can be calculated by n(a) = 1/(1 − Φ(a)).
Normal distribution
For the normal distribution (with median 0 and standard derivation 1, to keep things simple), we can look up the expected value of the one-sided truncated normal distribution and obtain
e(a) = E(X ∣ X > a) − a = φ(a)/(1 − Φ(a)) − a
Now is this growing or shrinking? We can plot this an have a quick look:
Indeed it is, too, a decreasing function!
(As a sanity check we can see that e(0) = √(2/π), which is the expected value of the half-normal distribution, as it should.)
Could it be any different?
This settles my question: It seems that each new surprisingly high water will tend to be less surprising than the previously – assuming high waters were uniformly or normally distributed, which is unlikely to be helpful.
This does raise the question, though, if there are probability distributions for which e(a) is be increasing?
I can try to construct one, and because it’s a bit easier, I’ll consider a discrete distribution on the positive natural numbers, and consider at g(0) = E(X) and g(1) = E(X − 1 ∣ X > 1). What does it take for g(1) > g(0)? Using E(X) = p + (1 − p)E(X ∣ X > 1) for p = P(X = 1) we find that in order to have g(1) > g(0), we need E(X) > 1/p.
This is plausible because we get equality when E(X) = 1/p, as it precisely the case for the geometric distribution. And it is also plausible that it helps if p is large (so that the next first record is likely just 1) and if, nevertheless, E(X) is large (so that if we do get an outcome other than 1, it’s much larger).
Starting with the geometric distribution, where P(X > n ∣ X ≥ n) = pn = p (the probability of again not rolling a six) is constant, it seems that these pn is increasing, we get the desired behavior. So let p1 < p2 < pn < … be an increasing sequence of probabilities, and define X so that P(X = n) = p1 ⋅ ⋯ ⋅ pn − 1 ⋅ (1 − pn) (imagine the die wears off and the more often you roll it, the less likely it shows a 6). Then for this variation of the game, every new record tends to exceed the previous more than previous records. As the p increase, we get a flatter long end in the probability distribution.
Gamma distribution
To get a nice plot, I’ll take the intuition from this and turn to continuous distributions. The Wikipedia page for the exponential distribution says it is a special case of the gamma distribution, which has an additional shape parameter α, and it seems that it could influence the shape of the distribution to be and make the probability distribution have a longer end. Let’s play around with β = 2 and α = 0.5, 1 and 1.5:
For α = 1 (dotted) this should just be the exponential distribution, and we see that e(a) is flat, as predicted earlier.
For larger α (dashed) the graph does not look much different from the one for the normal distribution – not a surprise, as for α → ∞, the gamma distribution turns into the normal distribution.
For smaller α (solid) we get the desired effect: e(a) is increasing. This means that new records tend to break records more impressively.
The orange line shows that this comes at a cost: for a given old record a, new records are harder to come by with smaller α.
Conclusion
As usual, it all depends on the distribution. Otherwise, not much, it’s late.
Blogging on Lean
This blog has become a bit quiet since I joined the Lean FRO. One reasons is of course that I can now improve things about Lean, rather than blog about what I think should be done (which, by contraposition, means I shouldn’t blog about what can be improved…). A better reason is that some of the things I’d otherwise write here are now published on the official Lean blog, in particular two lengthy technical posts explaining aspects of Lean that I worked on:
It would not be useful to re-publish them here because the technology verso
behind the Lean blog, created by my colleage David Thrane Christansen, enables such fancy features like type-checked code snippets, including output and lots of information on hover. So I’ll be content with just cross-linking my posts from here.
Convenient sandboxed development environment
I like using one machine and setup for everything, from serious development work to hobby projects to managing my finances. This is very convenient, as often the lines between these are blurred. But it is also scary if I think of the large number of people who I have to trust to not want to extract all my personal data. Whenever I run a cabal install
, or a fun VSCode extension gets updated, or anything like that, I am running code that could be malicious or buggy.
In a way it is surprising and reassuring that, as far as I can tell, this commonly does not happen. Most open source developers out there seem to be nice and well-meaning, after all.
Convenient or it won’t happen
Nevertheless I thought I should do something about this. The safest option would probably to use dedicated virtual machines for the development work, with very little interaction with my main system. But knowing me, that did not seem likely to happen, as it sounded like a fair amount of hassle. So I aimed for a viable compromise between security and convenient, and one that does not get too much in the way of my current habits.
For instance, it seems desirable to have the project files accessible from my unconstrained environment. This way, I could perform certain actions that need access to secret keys or tokens, but are (unlikely) to run code (e.g. git push
, git pull
from private repositories, gh pr create
) from “the outside”, and the actual build environment can do without access to these secrets.
The user experience I thus want is a quick way to enter a “development environment” where I can do most of the things I need to do while programming (network access, running command line and GUI programs), with access to the current project, but without access to my actual /home
directory.
I initially followed the blog post “Application Isolation using NixOS Containers” by Marcin Sucharski and got something working that mostly did what I wanted, but then a colleague pointed out that tools like firejail
can achieve roughly the same with a less “global” setup. I tried to use firejail
, but found it to be a bit too inflexible for my particular whims, so I ended up writing a small wrapper around the lower level sandboxing tool https://github.com/containers/bubblewrap.
Selective bubblewrapping
This script, called dev
and included below, builds a new filesystem namespace with minimal /proc
and /dev
directories, it’s own /tmp
directories. It then binds-mound some directories to make the host’s NixOS system available inside the container (/bin
, /usr
, the nix store including domain socket, stuff for OpenGL applications). My user’s home directory is taken from ~/.dev-home
and some configuration files are bind-mounted for convenient sharing. I intentionally don’t share most of the configuration – for example, a direnv enable
in the dev environment should not affect the main environment. The X11 socket for graphical applications and the corresponding .Xauthority
file is made available. And finally, if I run dev
in a project directory, this project directory is bind mounted writable, and the current working directory is preserved.
The effect is that I can type dev
on the command line to enter “dev mode” rather conveniently. I can run development tools, including graphical ones like VSCode, and especially the latter with its extensions is part of the sandbox. To do a git push
I either exit the development environment (Ctrl-D) or open a separate terminal. Overall, the inconvenience of switching back and forth seems worth the extra protection.
Clearly, isn’t going to hold against a determined and maybe targeted attacker (e.g. access to the X11 and the nix daemon socket can probably be used to escape easily). But I hope it will help against a compromised dev dependency that just deletes or exfiltrates data, like keys or passwords, from the usual places in $HOME
.
Rough corners
There is more polishing that could be done.
In particular, clicking on a link inside VSCode in the container will currently open Firefox inside the container, without access to my settings and cookies etc. Ideally, links would be opened in the Firefox running outside. This is a problem that has a solution in the world of applications that are sandboxed with Flatpak, and involves a bunch of moving parts (a xdg-desktop-portal user service, a filtering dbus proxy, exposing access to that proxy in the container). I experimented with that for a bit longer than I should have, but could not get it to work to satisfaction (even without a container involved, I could not get
xdg-desktop-portal
to heed my default browser settings…). For now I will live with manually copying and pasting URLs, we’ll see how long this lasts.With this setup (and unlike the NixOS container setup I tried first), the same applications are installed inside and outside. It might be useful to separate the set of installed programs: There is simply no point in running
evolution
orfirefox
inside the container, and if I do not even have VSCode orcabal
available outside, so that it’s less likely that I forget to enterdev
before using these tools.It shouldn’t be too hard to cargo-cult some of the NixOS Containers infrastructure to be able to have a separate system configuration that I can manage as part of my normal system configuration and make available to
bubblewrap
here.
So likely I will refine this some more over time. Or get tired of typing dev
and going back to what I did before…
The script
The dev
script (at the time of writing)
#!/usr/bin/env bash
extra=()
if [[ "$PWD" == /home/jojo/build/* ]] || [[ "$PWD" == /home/jojo/projekte/programming/* ]]
then
extra+=(--bind "$PWD" "$PWD" --chdir "$PWD")
fi
if [ -n "$1" ]
then
cmd=( "$@" )
else
cmd=( bash )
fi
# Caveats:
# * access to all of `/etc`
# * access to `/nix/var/nix/daemon-socket/socket`, and is trusted user (but needed to run nix)
# * access to X11
exec bwrap \
--unshare-all \
\
`# blank slate` \
--share-net \
--proc /proc \
--dev /dev \
--tmpfs /tmp \
--tmpfs /run/user/1000 \
\
`# Needed for GLX applications, in paticular alacritty` \
--dev-bind /dev/dri /dev/dri \
--ro-bind /sys/dev/char /sys/dev/char \
--ro-bind /sys/devices/pci0000:00 /sys/devices/pci0000:00 \
--ro-bind /run/opengl-driver /run/opengl-driver \
\
--ro-bind /bin /bin \
--ro-bind /usr /usr \
--ro-bind /run/current-system /run/current-system \
--ro-bind /nix /nix \
--ro-bind /etc /etc \
--ro-bind /run/systemd/resolve/stub-resolv.conf /run/systemd/resolve/stub-resolv.conf \
\
--bind ~/.dev-home /home/jojo \
--ro-bind ~/.config/alacritty ~/.config/alacritty \
--ro-bind ~/.config/nvim ~/.config/nvim \
--ro-bind ~/.local/share/nvim ~/.local/share/nvim \
--ro-bind ~/.bin ~/.bin \
\
--bind /tmp/.X11-unix/X0 /tmp/.X11-unix/X0 \
--bind ~/.Xauthority ~/.Xauthority \
--setenv DISPLAY :0 \
\
--setenv container dev \
"${extra[@]}" \
-- \
"${cmd[@]}"
GHC Steering Committee Retrospective
After seven years of service as member and secretary on the GHC Steering Committee, I have resigned from that role. So this is a good time to look back and retrace the formation of the GHC proposal process and committee.
In my memory, I helped define and shape the proposal process, optimizing it for effectiveness and throughput, but memory can be misleading, and judging from the paper trail in my email archives, this was indeed mostly Ben Gamari’s and Richard Eisenberg’s achievement: Already in Summer of 2016, Ben Gamari set up the ghc-proposals Github repository with a sketch of a process and sent out a call for nominations on the GHC user’s mailing list, which I replied to. The Simons picked the first set of members, and in the fall of 2016 we discussed the committee’s by-laws and procedures. As so often, Richard was an influential shaping force here.
Three ingredients
For example, it was him that suggested that for each proposal we have one committee member be the “Shepherd”, overseeing the discussion. I believe this was one ingredient for the process effectiveness: There is always one person in charge, and thus we avoid the delays incurred when any one of a non-singleton set of volunteers have to do the next step (and everyone hopes someone else does it).
The next ingredient was that we do not usually require a vote among all members (again, not easy with volunteers with limited bandwidth and occasional phases of absence). Instead, the shepherd makes a recommendation (accept/reject), and if the other committee members do not complain, this silence is taken as consent, and we come to a decision. It seems this idea can also be traced back on Richard, who suggested that “once a decision is requested, the shepherd [generates] consensus. If consensus is elusive, then we vote.”
At the end of the year we agreed and wrote down these rules, created the mailing list for our internal, but publicly archived committee discussions, and began accepting proposals, starting with Adam Gundry’s OverloadedRecordFields
.
At that point, there was no “secretary” role yet, so how I did become one? It seems that in February 2017 I started to clean-up and refine the process documentation, fixing “bugs in the process” (like requiring authors to set Github labels when they don’t even have permissions to do that). This in particular meant that someone from the committee had to manually handle submissions and so on, and by the aforementioned principle that at every step there ought to be exactly one person in change, the role of a secretary followed naturally. In the email in which I described that role I wrote:
Simon already shoved me towards picking up the “secretary” hat, to reduce load on Ben.
So when I merged the updated process documentation, I already listed myself “secretary”.
It wasn’t just Simon’s shoving that put my into the role, though. I dug out my original self-nomination email to Ben, and among other things I wrote:
I also hope that there is going to be clear responsibilities and a clear workflow among the committee. E.g. someone (possibly rotating), maybe called the secretary, who is in charge of having an initial look at proposals and then assigning it to a member who shepherds the proposal.
So it is hardly a surprise that I became secretary, when it was dear to my heart to have a smooth continuous process here.
I am rather content with the result: These three ingredients – single secretary, per-proposal shepherds, silence-is-consent – helped the committee to be effective throughout its existence, even as every once in a while individual members dropped out.
Ulterior motivation
I must admit, however, there was an ulterior motivation behind me grabbing the secretary role: Yes, I did want the committee to succeed, and I did want that authors receive timely, good and decisive feedback on their proposals – but I did not really want to have to do that part.
I am, in fact, a lousy proposal reviewer. I am too generous when reading proposals, and more likely mentally fill gaps in a specification rather than spotting them. Always optimistically assuming that the authors surely know what they are doing, rather than critically assessing the impact, the implementation cost and the interaction with other language features.
And, maybe more importantly: why should I know which changes are good and which are not so good in the long run? Clearly, the authors cared enough about a proposal to put it forward, so there is some need… and I do believe that Haskell should stay an evolving and innovating language… but how does this help me decide about this or that particular feature.
I even, during the formation of the committee, explicitly asked that we write down some guidance on “Vision and Guideline”; do we want to foster change or innovation, or be selective gatekeepers? Should we accept features that are proven to be useful, or should we accept features so that they can prove to be useful? This discussion, however, did not lead to a concrete result, and the assessment of proposals relied on the sum of each member’s personal preference, expertise and gut feeling. I am not saying that this was a mistake: It is hard to come up with a general guideline here, and even harder to find one that does justice to each individual proposal.
So the secret motivation for me to grab the secretary post was that I could contribute without having to judge proposals. Being secretary allowed me to assign most proposals to others to shepherd, and only once in a while myself took care of a proposal, when it seemed to be very straight-forward. Sneaky, ain’t it?
7 Years later
For years to come I happily played secretary: When an author finished their proposal and public discussion ebbed down they would ping me on GitHub, I would pick a suitable shepherd among the committee and ask them to judge the proposal. Eventually, the committee would come to a conclusion, usually by implicit consent, sometimes by voting, and I’d merge the pull request and update the metadata thereon. Every few months I’d summarize the current state of affairs to the committee (what happened since the last update, which proposals are currently on our plate), and once per year gathered the data for Simon Peyton Jones’ annually GHC Status Report. Sometimes some members needed a nudge or two to act. Some would eventually step down, and I’d sent around a call for nominations and when the nominations came in, distributed them off-list among the committee and tallied the votes.
Initially, that was exciting. For a long while it was a pleasant and rewarding routine. Eventually, it became a mere chore. I noticed that I didn’t quite care so much anymore about some of the discussion, and there was a decent amount of naval-gazing, meta-discussions and some wrangling about claims of authority that was probably useful and necessary, but wasn’t particularly fun.
I also began to notice weaknesses in the processes that I helped shape: We could really use some more automation for showing proposal statuses, notifying people when they have to act, and nudging them when they don’t. The whole silence-is-assent approach is good for throughput, but not necessary great for quality, and maybe the committee members need to be pushed more firmly to engage with each proposal. Like GHC itself, the committee processes deserve continuous refinement and refactoring, and since I could not muster the motivation to change my now well-trod secretarial ways, it was time for me to step down.
Luckily, Adam Gundry volunteered to take over, and that makes me feel much less bad for quitting. Thanks for that!
And although I am for my day job now enjoying a language that has many of the things out of the box that for Haskell are still only language extensions or even just future proposals (dependent types, BlockArguments
, do
notation with (← foo)
expressions and 💜 Unicode), I’m still around, hosting the Haskell Interlude Podcast, writing on this blog and hanging out at ZuriHac etc.
The Haskell Interlude Podcast
It was pointed out to me that I have not blogged about this, so better now than never:
Since 2021 I am – together with four other hosts – producing a regular podcast about Haskell, the Haskell Interlude. Roughly every two weeks two of us interview someone from the Haskell Community, and we chat for approximately an hour about how they came to Haskell, what they are doing with it, why they are doing it and what else is on their mind. Sometimes we talk to very famous people, like Simon Peyton Jones, and sometimes to people who maybe should be famous, but aren’t quite yet.
For most episodes we also have a transcript, so you can read the interviews instead, if you prefer, and you should find the podcast on most podcast apps as well. I do not know how reliable these statistics are, but supposedly we regularly have around 1300 listeners. We don’t get much feedback, however, so if you like the show, or dislike it, or have feedback, let us know (for example on the Haskell Disourse, which has a thread for each episode).
At the time of writing, we released 40 episodes. For the benefit of my (likely hypothetical) fans, or those who want to train an AI voice model for nefarious purposes, here is the list of episodes co-hosted by me:
- Gabriella Gonzales
- Jasper Van der Jeugt
- Chris Smith
- Sebastian Graf
- Simon Peyton Jones
- Ryan Trinkle
- Facundo Dominguez
- Marc Scholten
- Ben Gamari
- Andrew Lelechenko (Bodigrim)
- ZuriHac 2023 special
- Arnaud Spiwack
- John MacFarlane
- Moritz Angermann
- Jezen Thomas
Can’t decide where to start? The one with Ryan Trinkle might be my favorite.
Thanks to the Haskell Foundation and its sponsors for supporting this podcast (hosting, editing, transscription).
Joining the Lean FRO
Tomorrow is going to be a new first day in a new job for me: I am joining the Lean FRO, and I’m excited.
What is Lean?
Lean is the new kid on the block of theorem provers.
It’s a pure functional programming language (like Haskell, with and on which I have worked a lot), but it’s dependently typed (which Haskell may be evolving to be as well, but rather slowly and carefully). It has a refreshing syntax, built on top of a rather good (I have been told, not an expert here) macro system.
As a dependently typed programming language, it is also a theorem prover, or proof assistant, and there exists already a lively community of mathematicians who started to formalize mathematics in a coherent library, creatively called mathlib.
What is a FRO?
A Focused Research Organization has the organizational form of a small start up (small team, little overhead, a few years of runway), but its goals and measure for success are not commercial, as funding is provided by donors (in the case of the Lean FRO, the Simons Foundation International, the Alfred P. Sloan Foundation, and Richard Merkin). This allows us to build something that we believe is a contribution for the greater good, even though it’s not (or not yet) commercially interesting enough and does not fit other forms of funding (such as research grants) well. This is a very comfortable situation to be in.
Why am I excited?
To me, working on Lean seems to be the perfect mix: I have been working on language implementation for about a decade now, and always with a preference for functional languages. Add to that my interest in theorem proving, where I have used Isabelle and Coq so far, and played with Agda and others. So technically, clearly up my alley.
Furthermore, the language isn’t too old, and plenty of interesting things are simply still to do, rather than tried before. The ecosystem is still evolving, so there is a good chance to have some impact.
On the other hand, the language isn’t too young either. It is no longer an open question whether we will have users: we have them already, they hang out on zulip, so if I improve something, there is likely someone going to be happy about it, which is great. And the community seems to be welcoming and full of nice people.
Finally, this library of mathematics that these users are building is itself an amazing artifact: Lots of math in a consistent, machine-readable, maintained, documented, checked form! With a little bit of optimism I can imagine this changing how math research and education will be done in the future. It could be for math what Wikipedia is for encyclopedic knowledge and OpenStreetMap for maps – and the thought of facilitating that excites me.
With this new job I find that when I am telling friends and colleagues about it, I do not hesitate or hedge when asked why I am doing this. This is a good sign.
What will I be doing?
We’ll see what main tasks I’ll get to tackle initially, but knowing myself, I expect I’ll get broadly involved.
To get up to speed I started playing around with a few things already, and for example created Loogle, a Mathlib search engine inspired by Haskell’s Hoogle, including a Zulip bot integration. This seems to be useful and quite well received, so I’ll continue maintaining that.
Expect more about this and other contributions here in the future.
Squash your Github PRs with one click
TL;DR: Squash your PRs with one click at https://squasher.nomeata.de/.
Very recently I got this response from the project maintainer at a pull request I contributed: “Thanks, approved, please squash so that I can merge.”
It’s nice that my contribution can go it, but why did the maintainer not just press the “Squash and merge button”, and instead adds the this unnecessary roundtrip to the process? Anyways, maintainers make the rules, so I play by them. But unlike the maintainer, who can squash-and-merge with just one click, squashing the PR’s branch is surprisingly laberous: Github does not allow you to do that via the Web UI (and hence on mobile), and it seems you are expected to go to your computer and juggle with git rebase --interactive
.
I found this rather annoying, so I created Squasher, a simple service that will squash your branch for you. There is no configuration, just paste the PR url. It will use the PR title and body as the commit message (which is obviously the right way™), and create the commit in your name:
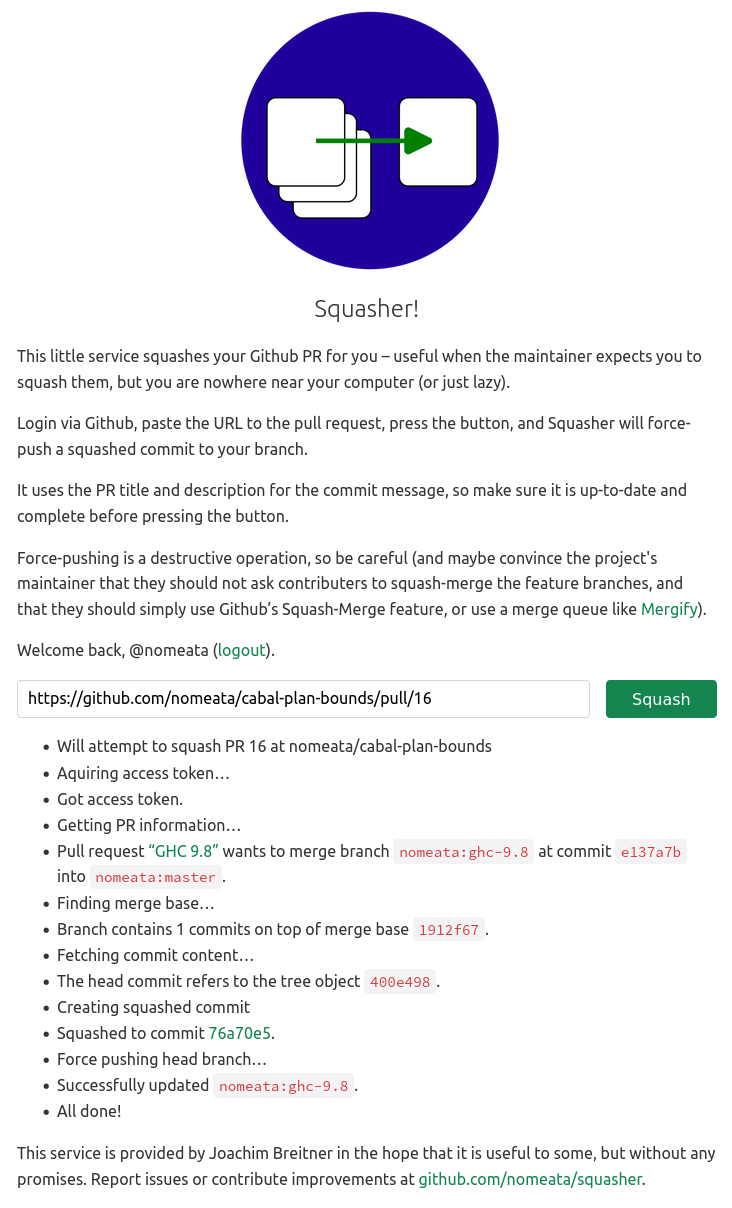
If you find this useful, or found it to be buggy, let me know. The code is at https://github.com/nomeata/squasher if you are curious about it.
Left recursive parser combinators via sharing
At this year’s ICFP in Seattle I gave a talk about my rec-def Haskell library, which I have blogged about before here. While my functional pearl paper focuses on a concrete use-case and the tricks of the implementation, in my talk I put the emphasis on the high-level idea: it beholds of a declarative lazy functional like Haskell that recursive equations just work whenever they describe a (unique) solution. Like in the paper, I used equations between sets as the running example, and only conjectured that it should also work for other domains, in particular parser combinators.
Naturally, someone called my bluff and asked if I actually tried it. I had not, but I should have, because it works nicely and is actually more straight-forward than with sets. I wrote up a prototype and showed it off a few days later as a lightning talk at Haskell Symposium; here is the write up that goes along with that.
Parser combinators
Parser combinators are libraries that provide little functions (combinators) that you compose to define your parser directly in your programming language, as opposed to using external tools that read some grammar description and generate parser code, and are quite popular in Haskell (e.g. parsec, attoparsec, megaparsec).
Let us define a little parser that recognizes sequences of a
s:
> let aaa = tok 'a' *> aaa <|> pure ()
ghci> parse aaa "aaaa"
ghciJust ()
> parse aaa "aabaa"
ghciNothing
Left-recursion
This works nicely, but just because we were lucky: We wrote the parser to recurse on the right (of the *>
), and this happens to work. If we put the recursive call first, it doesn’t anymore:
> let aaa = aaa <* tok 'a' <|> pure ()
ghci> parse aaa "aaaa"
ghci^CInterrupted.
This is a well-known problem (see for example Nicolas Wu’s overview paper), all the common parser combinator libraries cannot handle it and the usual advise is to refactor your grammar to avoid left recursion.
But there are some libraries that can handle left recursion, at least with a little help from the programmer. I found two variations:
The library provides an explicit fix point combinator, and as long as that is used, left-recursion works. This is for example described by Frost, Hafiz and Callaghan by, and (of course) Oleg Kiselyov has an implementation of this too.
The library expects explicit labels on recursive productions, so that the library can recognize left-recursion. I found an implementation of this idea in the
Agda.Utils.Parser.MemoisedCPS
module in the Agda code, thegll
library seems to follow this style and Jaro discusses it as well.
I took the module from the Agda source and simplified a bit for the purposes of this demonstration (Parser.hs
). Indeed, I can make the left-recursive grammar work:
> let aaa = memoise ":-)" $ aaa <* tok 'a' <|> pure ()
ghci> parse aaa "aaaa"
ghciJust ()
> parse aaa "aabaa"
ghciNothing
It does not matter what I pass to memoise
, as long as I do not pass the same key when memoising a different parser.
For reference, an excerpt of the the API of Parser
:
data Parser k tok a -- k is type of keys, tok type of tokens (e.g. Char)
instance Functor (Parser k tok)
instance Applicative (Parser k tok)
instance Alternative (Parser k tok)
instance Monad (Parser k tok)
parse :: Parser k tok a -> [tok] -> Maybe a
sat :: (tok -> Bool) -> Parser k tok tok
tok :: Eq tok => tok -> Parser k tok tok
memoise :: Ord k => k -> Parser k tok a -> Parser k tok a
Left-recursion through sharing
To follow the agenda set out in my talk, I now want to wrap that parser in a way that relieves me from having to insert the calls to memoise
. To start, I import that parser qualified, define a newtype around it, and start lifting some of the functions:
import qualified Parser as P
newtype Parser tok a = MkP { unP :: P.Parser Unique tok a }
parse :: Parser tok a -> [tok] -> Maybe a
MkP p) = P.parse p
parses (
sat :: Typeable tok => (tok -> Bool) -> Parser tok tok
= MkP (P.sat p)
sat p
tok :: Eq tok => tok -> Parser tok tok
= MkP (P.tok t) tok t
So far, nothing interesting had to happen, because so far I cannot build recursive parsers. The first interesting combinator that allows me to do that is <*>
from the Applicative
class, so I should use memoise
there. The question is: Where does the unique key come from?
Proprioception
As with the rec-def library, pure code won’t do, and I have to get my hands dirty: I really want a fresh unique label out of thin air. To that end, I define the following combinator, with naming aided by Richard Eisenberg:
propriocept :: (Unique -> a) -> a
= unsafePerformIO $ f <$> newUnique propriocept f
A thunk defined with propriocept
will know about it’s own identity, and will be able to tell itself apart from other such thunks. This gives us a form of observable sharing, precisely what we need. But before we return to our parser combinators, let us briefly explore this combinator.
Using propriocept
I can define an operation cons :: [Int] -> [Int]
that records (the hash of) this Unique
in the list:
> let cons xs = propriocept (\x -> hashUnique x : xs)
ghci> :t cons
ghcicons :: [Int] -> [Int]
This lets us see the identity of a list cell, that is, of the concrete object in memory.
Naturally, if we construct a finite list, each list cell is different:
> cons (cons (cons []))
ghci1,2,3] [
And if we do that again, we see that fresh list cells are allocated:
> cons (cons (cons []))
ghci4,5,6] [
We can create an infinite list; if we do it without sharing, every cell is separate:
> take 20 (acyclic 0)
ghci7,8,9,10,11,12,13,14,15,16,17,18,19,20,21,22,23,24,25,26] [
but if we tie the knot using sharing, all the cells in the list are actually the same:
> let cyclic = cons cyclic
ghci> take 20 cyclic
ghci27,27,27,27,27,27,27,27,27,27,27,27,27,27,27,27,27,27,27,27] [
We can achieve the same using fix
from Data.Function
:
> import Data.Function
ghci> take 20 (fix cons)
ghci28,28,28,28,28,28,28,28,28,28,28,28,28,28,28,28,28,28,28,28] [
I explore these heap structures more visually in a series of screencasts.
So with propriocept
we can distinguish different heap objects, and also recognize when we come across the same heap object again.
Left-recursion through sharing (cont.)
With that we return to our parser. We define a smart constructor for the new Parser
that passes the unique from propriocept
to the underlying parser’s memoise
function:
withMemo :: P.Parser Unique tok a -> Parser tok a
= propriocept $ \u -> MkP $ P.memoise u p withMemo p
If we now use this in the definition of all possibly recursive parsers, then the necessary calls to memoise
will be in place:
instance Functor (Parser tok) where
fmap f p = withMemo (fmap f (unP p))
instance Applicative (Parser tok) where
pure x = MkP (pure x)
<*> p2 = withMemo (unP p1 <*> unP p2)
p1
instance Alternative (Parser tok) where
= MkP empty
empty <|> p2 = withMemo (unP p1 <|> unP p2)
p1
instance Monad (Parser tok) where
return = pure
>>= f = withMemo $ unP p1 >>= unP . f p1
And indeed, it works (see RParser.hs
for the full code):
> let aaa = aaa <* tok 'a' <|> pure ()
ghci> parse aaa "aaaa"
ghciJust ()
> parse aaa "aabaa"
ghciNothing
A larger example
Let us try this on a larger example, and parse (simple) BNF grammars. Here is a data type describing them
type Ident = String
type RuleRhs = [Seq]
type Seq = [Atom]
data Atom = Lit String | NonTerm Ident deriving Show
type Rule = (Ident, RuleRhs)
type BNF = [Rule]
For the concrete syntax, I’d like to be able to parse something like
numExp :: String
= unlines
numExp "term := sum;"
[ "pdigit := '1' | '2' | '3' | '4' | '5' | '6' | '7' | '8' | '9';"
, "digit := '0' | pdigit;"
, "pnum := pdigit | pnum digit;"
, "num := '0' | pnum;"
, "prod := atom | atom '*' prod;"
, "sum := prod | prod '+' sum;"
, "atom := num | '(' term ')';"
, ]
so here is a possible parser; mostly straight-forward use of parser combinator:
type P = Parser Char
snoc :: [a] -> a -> [a]
= xs ++ [x]
snoc xs x
l :: P a -> P a
= p <|> l p <* sat isSpace
l p quote :: P Char
= tok '\''
quote quoted :: P a -> P a
= quote *> p <* quote
quoted p str :: P String
= some (sat (not . (== '\'')))
str ident :: P Ident
= some (sat (\c -> isAlphaNum c && isAscii c))
ident atom :: P Atom
= Lit <$> l (quoted str)
atom <|> NonTerm <$> l ident
eps :: P ()
= void $ l (tok 'ε')
eps sep :: P ()
= void $ some (sat isSpace)
sep sq :: P Seq
= [] <$ eps
sq <|> snoc <$> sq <* sep <*> atom
<|> pure <$> atom
ruleRhs :: P RuleRhs
= pure <$> sq
ruleRhs <|> snoc <$> ruleRhs <* l (tok '|') <*> sq
rule :: P Rule
= (,) <$> l ident <* l (tok ':' *> tok '=') <*> ruleRhs <* l (tok ';')
rule bnf :: P BNF
= pure <$> rule
bnf <|> snoc <$> bnf <*> rule
I somewhat sillily used snoc
rather than (:)
to build my lists, just so that I can show off all the left-recursion in this grammar.
Sharing is tricky
Let’s try it:
> parse bnf numExp
ghci^CInterrupted.
What a pity, it does not work! What went wrong?
The underlying library can handle left-recursion if it can recognize it by seeing a memoise
label passed again. This works fine in all the places where we re-use a parser definition (e.g. in bnf
), but it really requires that values are shared!
If we look carefully at our definition of l
(which parses a lexeme, i.e. something possibly followed by whitespace), then it recurses via a fresh function call, and the program will keep expanding the definition – just like the acyclic
above:
l :: P a -> P a
= p <|> l p <* sat isSpace l p
The fix (sic!) is to make sure that the recursive call is using the parser we are currently defining, which we can easily do with a local definition:
l :: P a -> P a
= p'
l p where p' = p <|> p' <* sat isSpace
With this little fix, the parser can parse the example grammar:
> parse bnf numExp
ghciJust [("term",[[NonTerm "sum"]]),("pdigit",[[Lit "1"],…
Going meta
The main demonstration is over, but since we now have already have a parser for grammar descriptions at hand, let’s go a bit further and dynamically construct a parser from such a description. The parser should only accept strings according to that grammar, and return a parse tree annotated with the non-terminals used:
interp :: BNF -> P String
= parsers M.! start
interp bnf where
start :: Ident
= fst (head bnf)
start
parsers :: M.Map Ident (P String)
= M.fromList [ (i, parseRule i rhs) | (i, rhs) <- bnf ]
parsers
parseRule :: Ident -> RuleRhs -> P String
= trace <$> asum (map parseSeq rhs)
parseRule ident rhs where trace s = ident ++ "(" ++ s ++ ")"
parseSeq :: Seq -> P String
= fmap concat . traverse parseAtom
parseSeq
parseAtom :: Atom -> P String
Lit s) = traverse tok s
parseAtom (NonTerm i) = parsers M.! i parseAtom (
Let’s see it in action (full code in BNFEx.hs
):
> Just bnfp = parse bnf numExp
ghci> :t bnfp
ghcibnfp :: BNF
> parse (inter
ghciinteract interp
> parse (interp bnfp) "12+3*4"
ghciJust "term(sum(prod(atom(num(pnum(pnum(pdigit(1))digit(pdigit(2))))))+sum(prod(atom(num(pnum(pdigit(3))))*prod(atom(num(pnum(pdigit(4)))))))))"
> parse (interp bnfp) "12+3*4+"
ghciNothing
It is worth noting that the numExp
grammar is also left-recursive, so implementing interp
with a conventional parser combinator library would not have worked. But thanks to our propriocept
tick, it does! Again, the sharing is important; in the code above it is the map parsers
that is defined in terms of itself, and will ensure that the left-recursive productions will work.
Closing thoughts
I am using unsafePerformIO
, so I need to justify its use. Clearly, propriocept
is not a pure function, and it’s type is a lie. In general, using it will break the nice equational properties of Haskell, as we have seen in our experiments with cons
.
In the case of our parser library, however, we use it in specific ways, namely to feed a fresh name to memoise
. Assuming the underlying parser library’s behavior does not observably depend on where and with which key memoise
is used, this results in a properly pure interface, and all is well again. (NB: I did not investigate if this assumption actually holds for the parser library used here, or if it may for example affect the order of parse trees returned.)
I also expect that this implementation, which will memoise every parser involved, will be rather slow. It seems plausible to analyze the graph structure and figure out which memoise
calls are actually needed to break left-recursion (at least if we drop the Monad
instance or always memoise >>=
).
If you liked this post, you might enjoy reading the paper about rec-def, watch one of my talks about it (MuniHac, BOBKonf, ICFP23; the presentation evolved over time), or if you just want to see more about how things are laid out on Haskell’s heap, go to my screen casts exploring the Haskell heap.
Generating bibtex bibliographies from DOIs via DBLP
I sometimes write papers and part of paper writing is assembling the bibliography. In my case, this is done using BibTeX. So when I need to add another citation, I have to find suitable data in Bibtex format.
Often I copy snippets from .bib
files from earlier paper.
Or I search for the paper on DBLP, which in my experience has highest quality BibTeX entries and best coverage of computer science related publications, copy it to my .bib
file, and change the key to whatever I want to refer the paper by.
But in the days of pervasive use of DOIs (digital object identifiers) for almost all publications, manually keeping the data in bibtex files seems outdated. Instead I’d rather just put the two pieces of data I care about: the key that I want to use for citation, and the doi. The rest I do not want to be bothered with.
So I wrote a small script that takes a .yaml
file like
entries:
unsafePerformIO: 10.1007/10722298_3
dejafu: 10.1145/2804302.2804306
runST: 10.1145/3158152
quickcheck: 10.1145/351240.351266
optimiser: 10.1016/S0167-6423(97)00029-4
sabry: 10.1017/s0956796897002943
concurrent: 10.1145/237721.237794
launchbury: 10.1145/158511.158618
datafun: 10.1145/2951913.2951948
observable-sharing: 10.1007/3-540-46674-6_7
kildall-73: 10.1145/512927.512945
kam-ullman-76: 10.1145/321921.321938
spygame: 10.1145/3371101
cocaml: 10.3233/FI-2017-1473
secrets: 10.1017/S0956796802004331
modular: 10.1017/S0956796817000016
longley: 10.1145/317636.317775
nievergelt: 10.1145/800152.804906
runST2: 10.1145/3527326
polakow: 10.1145/2804302.2804309
lvars: 10.1145/2502323.2502326
typesafe-sharing: 10.1145/1596638.1596653
pure-functional: 10.1007/978-3-642-14162-1_17
clairvoyant: 10.1145/3341718
subs:
- replace: Peyton Jones
with: '{Peyton Jones}'
and turns it into a nice .bibtex
file:
$ ./doi2bib.py < doibib.yaml > dblp.bib
$ head dblp.bib
@inproceedings{unsafePerformIO,
author = {Simon L. {Peyton Jones} and
Simon Marlow and
Conal Elliott},
editor = {Pieter W. M. Koopman and
Chris Clack},
title = {Stretching the Storage Manager: Weak Pointers and Stable Names in
Haskell},
booktitle = {Implementation of Functional Languages, 11th International Workshop,
IFL'99, Lochem, The Netherlands, September 7-10, 1999, Selected Papers},
The last bit allows me to do some fine-tuning of the file, because unfortunately, not even DBLP BibTeX files are perfect, for example in the presence of two family names.
Now I have less moving parts to worry about, and a more consistent bibliography.
The script is rather small, so I’ll just share it here:
#!/usr/bin/env python3
import sys
import yaml
import requests
import requests_cache
import re
='sqlite')
requests_cache.install_cache(backend
= yaml.safe_load(sys.stdin)
data
for key, doi in data['entries'].items():
= requests.get(f"https://dblp.org/doi/{doi}.bib").text
bib = re.sub('{DBLP.*,', '{' + key + ',', bib)
bib for subs in data['subs']:
= re.sub(subs['replace'], subs['with'], bib)
bib print(bib)
There are similar projects out there, e.g. dblpbibtex
in C++ and dblpbib
in Ruby. These allow direct use of \cite{DBLP:rec/conf/isit/BreitnerS20}
in Latex, which is also nice, but for now I like to choose more speaking citation keys myself.
ICFP Pearl preprint on rec-def
I submitted a Functional Pearl to this year’s ICFP and it got accepted!
It is about the idea of using Haskell’s inherent ability to define recursive equations, and use them for more than just functions and lazy data structures. I blogged about this before (introducing the idea, behind the scenes, applications to program analysis, graph algorithms and minesweeper), but hopefully the paper brings out the idea even more clearly. The constructive feedback from a few friendly readers (Claudio, Sebastian, and also the anonymous reviewers) certainly improved the paper.
Abstract
Haskell’s laziness allows the programmer to solve some problems naturally and declaratively via recursive equations. Unfortunately, if the input is “too recursive”, these very elegant idioms can fall into the dreaded black hole, and the programmer has to resort to more pedestrian approaches.
It does not have to be that way: We built variants of common pure data structures (Booleans, sets) where recursive definitions are productive. Internally, the infamous unsafePerformIO is at work, but the user only sees a beautiful and pure API, and their pretty recursive idioms – magically – work again.
If you are curious, please have a look at the current version of the paper. Any feedback is welcome; even more if it comes before July 11, because then I can include it in the camera ready version.
There are still some open questions around this work. What bothers me maybe most is the lack of a denotational semantics that unifies the partial order underlying the Haskell fragment, and the partial order underlying the domain of the embedded equations.
The crux of the probem is maybe best captured by this question:
Imagine an untyped lambda calculus with constructors, lazy evaluation, and an operation
rseq
that recursively evaluates constructors, but terminates in the presence of cycles. So for examplerseq (let x = 1 :: x in x ) ≡ () rseq (let x () = 1 :: x () in x ()) ≡ ⊥
In this language, knot tying is observable. What is the “nicest” denotational semantics.
Update: I made some progress via a discussion on the Haskell Discource and started some rough notes on a denotational semantics.